Biography
Medicine and engineering run in Dr. Jeremias Sulam’s family. His mother is a physician, his father is a civil engineer, and his younger brother a mechanical engineer. In his early years, Dr. Sulam liked hearing about his mother’s work—especially when she described the challenging cases she faced as an anesthesiologist. He thought hard about becoming a doctor like his mother but, unsure on his ability to care for patients directly, Dr. Sulam created a way to merge both medicine and engineering: he became a biomedical engineer.
Dr. Sulam was born in Argentina. He grew up in Santa Fe, a mid-sized city about five hours north of Buenos Aires. He was a good student throughout his school career, and he balanced his studies with swimming and playing the piano. He also developed a love of math. In his senior year of high school, Dr. Sulam spent time in New Zealand as an exchange student, staying in a small town in the Waikato region, learning from and connecting with people who became influential in his life.
After high school, Dr. Sulam attended Universidad Nacional de Entre Ríos, a small university in Argentina renowned for its biomedical engineering program. He then went on to receive his Ph.D. in computer science from Technion - Israel Institute of Technology, working in signal and image processing, and machine learning. His Ph.D. advisor, Michael “Miki” Elad, was a big influence in Dr. Sulam’s life, influencing not just his technical repertoire
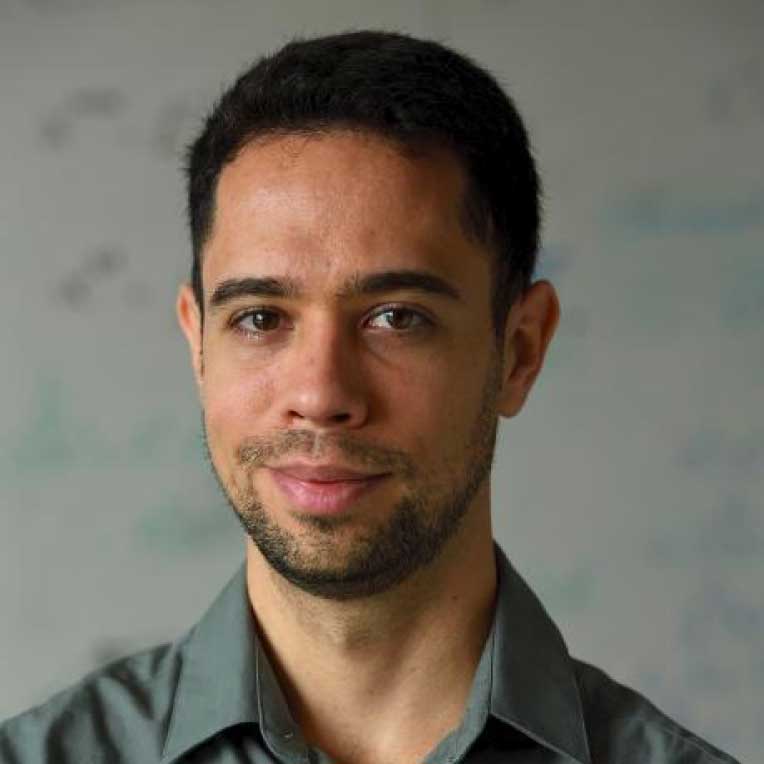
but his approach to dealing with problems more broadly. Using some of Miki’s analytical tools, Dr. Sulam’s thesis examined the use of sparse representation analysis to analyze deep learning models – a family of methods that have become very popular in machine learning and artificial intelligence.
Today, Dr. Jeremias Sulam is an assistant professor in the Department of Biomedical Engineering at Johns Hopkins University. He is also affiliated with the Mathematical Institute for Data Science, the Center for Imaging Science and the Kavli Neuroscience Discovery Institute. His team investigates the foundations of machine learning as well as its application to several problems in biomedical imaging.
“Did I really know what biomedical engineering was about when I was younger? As with most things in life, I had a rough understanding that changed over time. I just knew that I was interested in medical problems, I knew I was relatively good at math, and I enjoyed solving problems that had some analytical component to them. Biomedical engineering was the natural intersection of those disciplines.”
– Dr. Jeremias Sulam
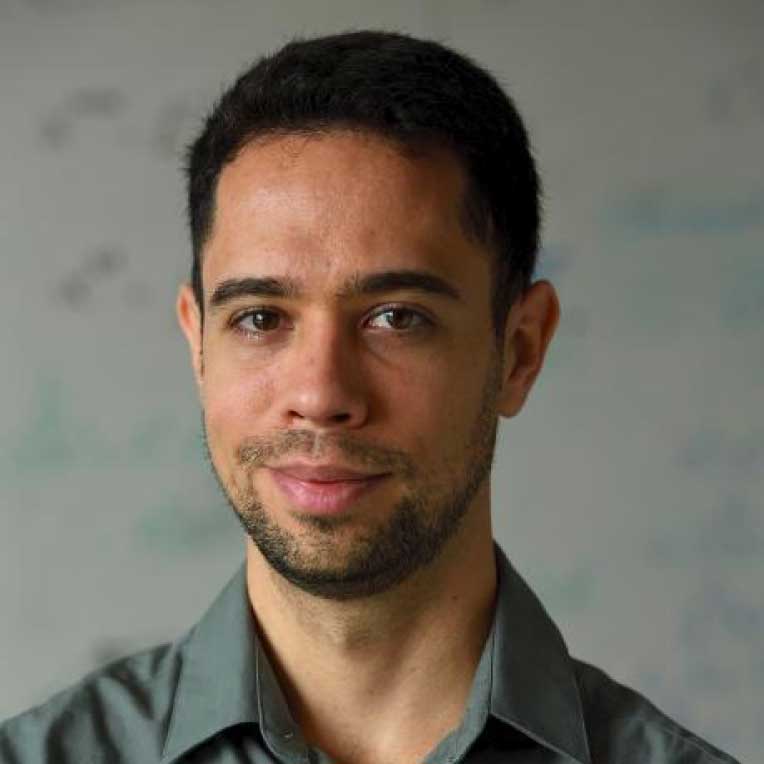
Biography
Medicine and engineering run in Dr. Jeremias Sulam’s family. His mother is a physician, his father is a civil engineer, and his younger brother a mechanical engineer. In his early years, Dr. Sulam liked hearing about his mother’s work—especially when she described the challenging cases she faced as an anesthesiologist. He thought hard about becoming a doctor like his mother but, unsure on his ability to care for patients directly, Dr. Sulam created a way to merge both medicine and engineering: he became a biomedical engineer.
Dr. Sulam was born in Argentina. He grew up in Santa Fe, a mid-sized city about five hours north of Buenos Aires. He was a good student throughout his school career, and he balanced his studies with swimming and playing the piano. He also developed a love of math. In his senior year of high school, Dr. Sulam spent time in New Zealand as an exchange student, staying in a small town in the Waikato region, learning from and connecting with people who became influential in his life.
After high school, Dr. Sulam attended Universidad Nacional de Entre Ríos, a small university in Argentina renowned for its biomedical engineering program. He then went on to receive his Ph.D. in computer science from Technion - Israel Institute of Technology, working in signal and image processing, and machine learning. His Ph.D. advisor, Michael “Miki” Elad, was a big influence in Dr. Sulam’s life, influencing not just his technical repertoire but his approach to dealing with problems more broadly. Using some of Miki’s analytical tools, Dr. Sulam’s thesis examined the use of sparse representation analysis to analyze deep learning models – a family of methods that have become very popular in machine learning and artificial intelligence.
Today, Dr. Jeremias Sulam is an assistant professor in the Department of Biomedical Engineering at Johns Hopkins University. He is also affiliated with the Mathematical Institute for Data Science, the Center for Imaging Science and the Kavli Neuroscience Discovery Institute. His team investigates the foundations of machine learning as well as its application to several problems in biomedical imaging.
“Did I really know what biomedical engineering was about when I was younger? As with most things in life, I had a rough understanding that changed over time. I just knew that I was interested in medical problems, I knew I was relatively good at math, and I enjoyed solving problems that had some analytical component to them. Biomedical engineering was the natural intersection of those disciplines.”
– Dr. Jeremias Sulam
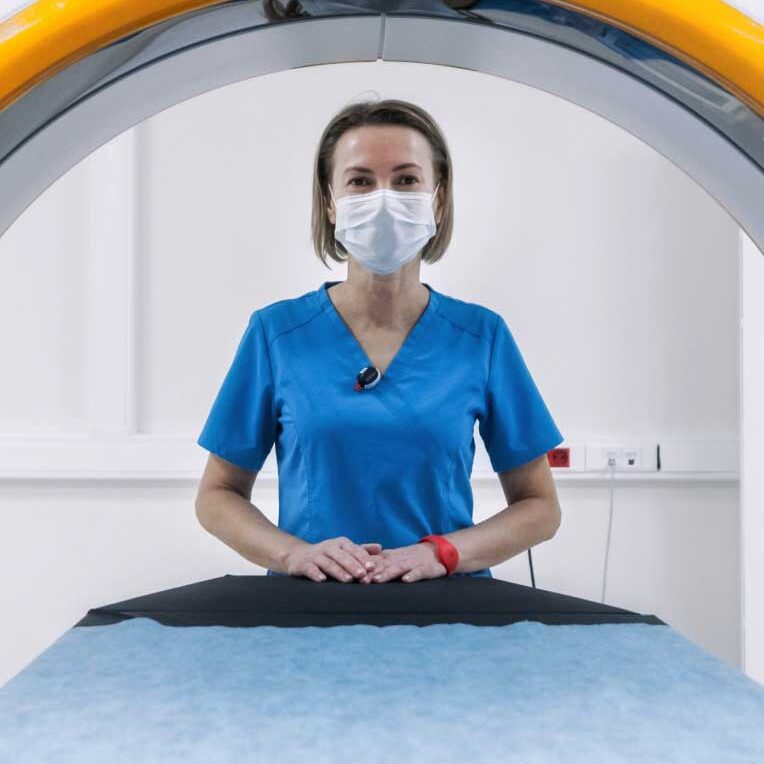
Research Focus
Challenges
While the explosion of machine learning and artificial intelligence is increasingly changing our world (from recommendation systems to autonomous vehicles), there’s still much to investigate on how to best leverage these advances to improve the ways in which we measure and image the human brain.
Tracking and mapping the main neuronal pathways in the brain is of critical value for understanding how the brain functions and develops, as well as for diagnosing and treating neurodegenerative diseases. The standard way to image these axon bundles in humans is via diffusion magnetic resonance imaging (MRI), relying mostly on the diffusion of water molecules. However, diffusion MRI suffers from poor spatial resolution, and is limited in the information that it can provide, particularly as it relates to neurodegenerative processes. A promising complement to diffusion-based approaches is Susceptibility Tensor Imaging (STI), which tries to quantify the amount, and direction, of magnetic susceptibility in the brain. Unfortunately, the protocols that are needed to reconstruct STI images are far from practical in the clinic, and, as a result, STI’s potential to understand the human brain remains underexplored.
Focus and Priorities
Dr. Sulam and his team are developing algorithms that can adapt from data and learn to solve these hard reconstruction problems more efficiently. To bring magnetic susceptibility-based imaging of the brain to be deployed in humans in vivo, Dr. Sulam and his team use tools based on deep neural networks and modern machine learning techniques to alleviate the shortcomings of current acquisition protocols. Better and easier protocols imply a more comfortable experience for the patient and a shorter examination period, opening the door to deploying these promising techniques in the clinic.
Focus and Priorities
Dr. Sulam and his team are developing algorithms that can adapt from data and learn to solve these hard reconstruction problems more efficiently. To bring magnetic susceptibility-based imaging of the brain to be deployed in humans in vivo, Dr. Sulam and his team use tools based on deep neural networks and modern machine learning techniques to alleviate the shortcomings of current acquisition protocols. Better and easier protocols imply a more comfortable experience for the patient and a shorter examination period, opening the door to deploying these promising techniques in the clinic.
More generally, Dr. Sulam’s team is interested in understanding when computational algorithms can be designed to solve hard problems – like the problem of brain imaging based on STI. Dr. Sulam’s research is driven by developing efficient methods, while studying ways to turn complex algorithms more interpretable and robust.
More generally, Dr. Sulam’s team is interested in understanding when computational algorithms can be designed to solve hard problems – like the problem of brain imaging based on STI. Dr. Sulam’s research is driven by developing efficient methods, while studying ways to turn complex algorithms more interpretable and robust.
Benefits
The physical principles behind STI are quite different from those that most other imaging modalities in MRI employ. In particular, since the magnetic susceptibility of brain tissue is affected to a large degree by the myelin sheaths that wrap around axon fibers, STI holds significant promise for the study of brain development and neurodegenerative diseases that are strongly associated with changes in myelin content. Dr. Sulam is optimistic that his team’s algorithms for STI reconstruction will one day enable the deployment of these techniques at scale in the clinical practice.
Karen Toffler Charitable Trust Investment
Support from the Karen Toffler Charitable Trust Investment will help Dr. Sulam continue the development and validation of their machine learning tools for susceptibility tensor imaging, bringing it one step closer to the use in humans in vivo. The grant will provide funds for his graduate students and researchers, as well as for the experimental validation of their results in animal models.
The Toffler Scholar Program will also importantly enable Dr. Sulam to bolster his scientific network, as he is now part of a community of scientists that connect, collaborate and support each other in their scholarly pursuits.
Karen Toffler Charitable Trust Investment
Support from the Karen Toffler Charitable Trust Investment will help Dr. Sulam continue the development and validation of their machine learning tools for susceptibility tensor imaging, bringing it one step closer to the use in humans in vivo. The grant will provide funds for his graduate students and researchers, as well as for the experimental validation of their results in animal models.
The Toffler Scholar Program will also importantly enable Dr. Sulam to bolster his scientific network, as he is now part of a community of scientists that connect, collaborate and support each other in their scholarly pursuits.
“We’ve learned a lot about what we can do when we have algorithms that learn directly from data and come up with solutions to certain hard problems. This has opened a whole set of possibilities in many different domains. What I am doing now is just bringing a little bit of these tools to the MRI community to solve this problem of susceptibility tensor imaging.”
– Dr. Jeremias Sulam